By Jerry Radich
Relapse is the bane of cancer therapy. Despite advances in understanding the genetics of cancer, targeted therapy, and immunological measures, relapse and resistance is an all-too-common outcome. Response to therapy follows one of the curves shown in Figure 1. The top curve shows refractory disease. The bottom curve shows a deep response (“cure”). The middle curve is the common outcome of patients enjoying an initial response to therapy, only to then relapse.*
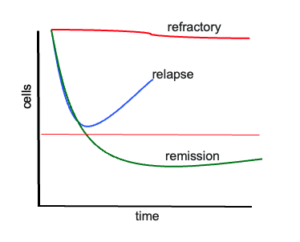
Why is the middle curve so prevalent? We used to think cancer occurred after a serial accumulation of mutations affecting different biological pathways. Once a critical number of mutations occurred, cancer was born. This massive proliferation was thought to create a genetic monolith composed of billions and billions of genetically alike cancer cells. This model can explain the two extreme cases of disease response, refractory or cure, depending on an individual’s luck of having a responsive or unresponsive genotype. The middle curve—response then relapse—is harder to explain with this model. We now know that cancer is made of many related, but not identical clones. Thus, as therapy begins, sensitive clones are eliminated, but this allows more room and food for resistant clones to grow. Quite simply, the main clone at diagnosis may not be the clone that eventually kills the patient. Taken this view, relapse is simply a normal byproduct of simple Darwinian selection: our best-intentioned treatment selects for drug-resistant cancer cells.**
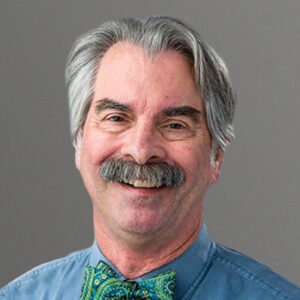
When patients relapse, it is usually more difficult to get them into a subsequent deep response, and relapse usually occurs more quickly. I often tell patients that treating their disease is akin to pests and pesticides. The metaphor is apparently much stronger than I thought. I just read a review by my friend and colleague Carlo Maley and his team. Their paper outlines the marked similarity between the issues facing farmers and oncologists in dealing with resistance.1 Insects share with cancer cells similar mechanisms of treatment resistance, including mutations to critical target genes, alteration of drug metabolism, and changes in drug uptake and exclusion. Decades of experience of managing insect pests to optimize crop health have led to the rules and principles that comprise what is called “integrated pest management” (IPM). These include the following:
1. Prevention. In farming, this can mean adjusting crops and the environment to be less friendly to pests. Prevention of human cancer is the logical emphasis of epidemiologists and sound public health policy (excepting the current tide of governmental shenanigans).
2. Predict response/resistance. In farming and cancer, any early indicators of pest/cancer behavior can inform treatment diseases. In cancer we have a variety of biomarkers for risk stratification, but these are very imperfect and often fail to work at the individual patient level. Oncology has much room for improvement here, as this holds great promise in guiding optimal choice of initial therapy, critical in minimizing the risk of resistance.
3. Preferentially choose nonchemical control. In farming this can be done with local environmental adjustments. In cancer this approach has limitations. In solid tumors, certainly surgical removal of the tumor fits the bill. In leukemia, CAR-T therapy is conceptually nonchemical, though resistance is still an issue. Perhaps research on manipulating the cancer microenvironment will best fit this principle.
4. Use target-specific drugs. Pesticides act on specific biological pathways, often not critical to plants (an example is organophosphates which block cholinesterase, blocking nerve activity in insects). In cancer, precision medicine is based on targeted essential mutated genes. CML is the obvious model with the tyrosine kinase inhibitor (TKI) therapy. At this point, however, the number of identified genetic mutations in cancer far outstrips the number of targeted agents. Pharmaceutical companies will not be running out of work for some time.
5. Use the lowest effective dose. Higher drug doses create more potential harm and complications in the environment (farming) and the patient (medicine). However, practical experience in the field and lab suggest higher doses are also more likely to select for resistance. CML again offers an example where patients with deep molecular response, even with detectable disease, can have their TKI therapy discontinued without subsequent relapse.
6. Prevent/reduce cross-resistance. Data in bugs and patients show that resistance occurs more often and faster when only one treatment agent is used. Using multiple agents with different mechanisms of action decreases and delays resistance (though in farming and cancer, resistance occurs even with multiple agent regimens). In farming, this principle calls for separating the application of treatment with rotations of agents with different mechanisms of action.
7. Monitor continuously. In farming and cancer therapy, monitoring allows for a measurement of response, prediction of future decline or increase, and characterization of resistance features (new mutations). Once again, CML is the first and best model, as peripheral blood measurement of BCR::ABL1 levels predicts long-term response and prompts resistance studies for ABL1 mutations if disease burden milestones are not hit.
8. Change interventions in response to burden. Frequent monitoring allows for early intervention if resistance is identified. Intervention may preclude resistance/relapse in some cases, especially when resistance is identified that is susceptible to a specific different targeted compound (again, think resistance ABL mutations in CML that are sensitive to a different TKI).
9. Identify minimum disease burden levels for treatment. This is to establish a threshold below which intervention may not be needed. An example would be the discontinuation of therapy when patients with leukemia have no evidence of measurable residual disease. The best example would be the discontinuation to patients with deep molecular response in CML.
10. Evaluate success based on long-term management. In farming, the long view means evaluation of pest control strategies based on year-after-year crop yields. In cancer, this emphasizes outcomes like overall survival and quality of life.
The authors go on to outline the implications in trial design and treatment (“IPM-inspired therapy”), using colon cancer as a model. These are easily imagined in the hematological malignancy arena. The key change in philosophy from the farm is the assumption that resistance clones are likely present from the beginning, and the optimal management embraces controlling the cancer, rather than trying to eliminate all cancerous cells (in CML this is often referred to as “a functional cure.”). Essentially, this means a therapeutic strategy that includes as an option living with low levels of cancer, rather than striving for absolute eradication of all cancer cells (indeed, it may be impossible in nonsurgical settings to completely eradicate all cancer, since if at diagnosis AML has ~1012 cells, getting to an MRD-negative state could leave the patient with at least a million AML cells).
An IPM approach would require overcoming several practical and scientific hurdles, requiring advances in our technology and knowledge base. First, we need more sensitive and cost-effective methods to monitor patients. Monitoring is the linchpin for the IPM approach, so we need to understand how frequently to monitor, where to monitor (blood, marrow or cell-free nucleic acid?), and how to monitor (eg, flow cytometry or sequencing based?). Plus, none of these issues matter if the monitoring test is not affordable for the frequency of testing required. Excellent monitoring and long-term outcome data will be essential to determining lower thresholds of disease burden for potential intervention discontinuation (or postponement). The IPM approach will need more selective, targeted agents that can be rotated often to prevent the selection of resistant populations. Lastly, we will need studies of clonal heterogeneity and selection—presumably the cancer is made of populations that compete, and others that cooperated. We need to know enough about the patient’s “cancer ecosystem” that we can therapeutically manipulate the system to give a competitive advantage to low aggressive clones that will not kill the patient.
Currently, cancer uses Darwinian selection against us. It’s time we use the laws of Darwin against cancer. Maybe it’s time to learn lessons from the land.
Notes
* The “width” of the curve (% if cases in each category) depends on the particular disease and state of remission/relapse. For newly diagnosed CML, the bottom good- response curve would be very thick compared to the other curves, while for relapsed/refractory AML, the middle and bottom curves would be wide and the lower curve quite thin.
** Another reason patients may respond then relapse arises from incomplete therapy—either not intense enough or not long enough. This is akin to culling the herd. In this situation, one might expect the genotype of the relapse to be the same as the diagnostic sample.
Reference
1. Seyedi S, Harris VK, Kapsetaki SE, et al. Resistance management for cancer: lessons from farmers. Cancer Res. 2024;84(22):3715-3727. doi:10.1158/0008-5472.CAN-23-3374
Jerry Radich is a professor at the Fred Hutchinson Cancer Center and an associate editor of SOHO Insider. Email [email protected].